Publications Hub, EuroPerio, Treatment, Article
How can periodontal treatment best be personalized?
12 May 2025
So-called P4 (predictive, preventive, personalized, participatory) healthcare has been evolving in medicine and in periodontology, where knowledge about general and local risk factors combined with advances in diagnostics and treatment are enabling the personalization of treatment and the individualization of periodontal care. A joint session by the EFP and the International Academy of Periodontology (IAP) at EuroPerio11 will consider various ways in which periodontal treatment can be personalized. Magda Feres describes advances in personalizing anti-microbial periodontal therapy, Alpdogan Kantarci outlines the "paradigm shift" needed to personalize anti-inflammatory periodontal therapy, and Christoph Ramseier explores how secondary prevention can be personalized.
How AI can be used to personalize antimicrobial periodontal treatment
By Magda Feres
Traditionally, antimicrobial periodontal treatment has followed an evidence-based framework, relying on randomized controlled trials (RCTs) and systematic reviews that focus on population-level outcomes. However, with the advent of machine learning, we can now uncover implicit patterns and relationships within data, enabling the development of tailored therapies based on an individual patient’s unique characteristics. This represents a transformative shift in treatment strategies.
In my presentation at EuroPerio11, I will introduce an AI-driven model capable of predicting patient responses to antimicrobial periodontal treatment[1]. This is the first prognostic model for individualized periodontal treatment response trained and tested on well-defined granular datasets from eight RCTs conducted across three continents. Our model was trained on 18 baseline features, including demographic, clinical, microbiological, and treatment-related parameters.
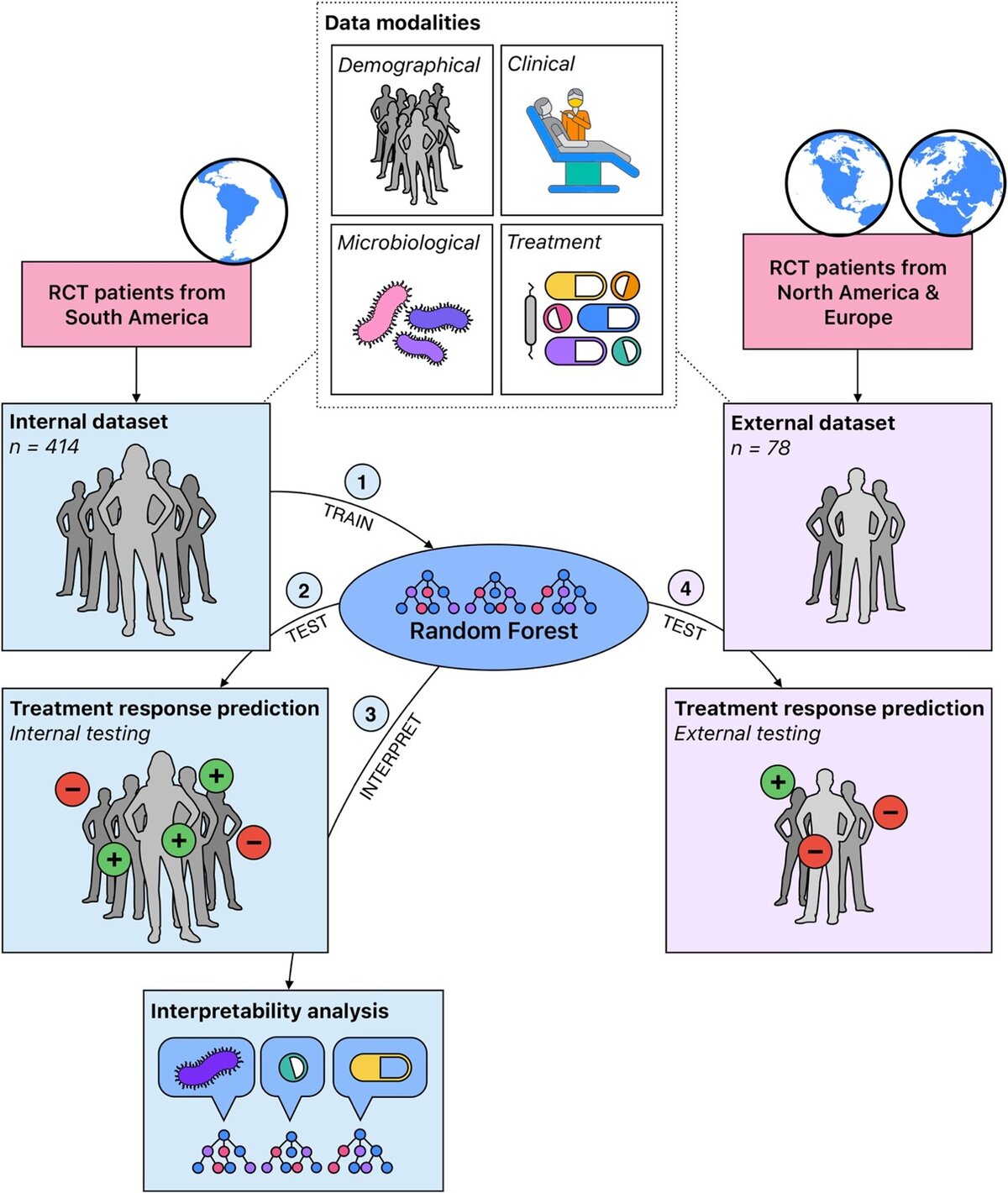
A key finding from the machine-learning analysis was its ability to reveal nuanced interactions between these variables—patterns that traditional statistical methods did not capture in our original RCTs. This AI-driven approach marks a significant step towards precision periodontology.
The future integration of this or similar prognostic models into clinical workflows via clinician-facing software holds significant promise for optimizing periodontal treatment decisions. These models can assist clinicians in selecting the most appropriate treatment strategy for each patient by generating individualized predictions for various therapeutic options—including antimicrobial agents with optimized dosages and durations, host-modulation therapies, and surgical interventions.
This approach allows clinicians to adopt the least intensive—yet most effective—treatment, which will reduce drug overuse, avoid unnecessary surgical interventions, and maximize treatment success.
[1] Feher B, de Souza Oliveira EH, Mendes Duarte P, Werdich AA, Giannobile WV, Feres M. Machine learning-assisted prediction of clinical responses to periodontal treatment. J Periodontol. 2025 Apr 20. doi: 10.1002/JPER.24-0737. Epub ahead of print. PMID: 40254962.
A paradigm shift is needed in anti-inflammatory periodontal therapy
By Alpdogan Kantarci
The resolution of inflammation is an active and receptor-mediated process, involving all cells of the immune system and structural cells. As opposed to the blockage of the inflammatory mechanisms—which also turns off the resolution pathways while stopping the activation—the resolution of the inflammation is driven by the active involvement of immune cells.
This requires a paradigm shift in our understanding of therapeutic approaches targeted for inflammatory diseases, including periodontal treatment. Active mediators of resolution are involved in the reprogramming of the inflammatory process, eliminating the ecological milieu for the growth and colonization of the microbial species in the periodontal pocket. This directly activates the periodontal ligament stem cells for wound healing and the regeneration of periodontal tissues, and restores the homeostatic balance to sustain the outcomes of treatment.
This novel approach therefore provides strong evidence for an understanding of periodontal therapy, which, by definition, will be customized for a personal treatment to focus on the individual’s cellular and molecular processes to target healing pathways.
Personalizing secondary prevention of periodontitis
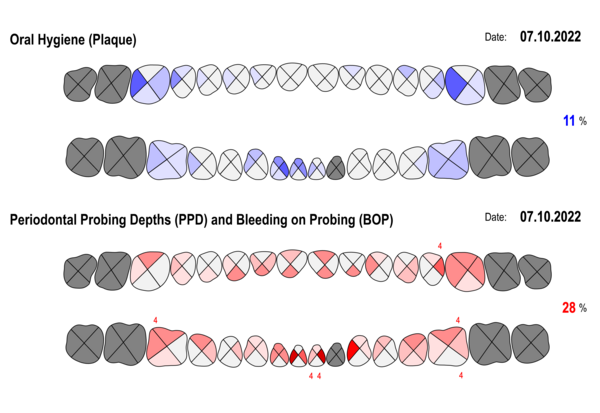
By Christoph Ramseier
Secondary prevention through supportive periodontal care (SPC) plays a key role in preventing disease recurrence after active periodontal treatment. However, current SPC protocols are still largely based on fixed recall intervals and generic recommendations. In the era of precision medicine, there is a growing consensus that a personalized approach could significantly improve long-term outcomes.
Personalization in secondary prevention is still in its infancy. While risk-based recall strategies have been proposed for many years, their implementation remains limited because of practical challenges in risk assessment and patient adherence. Recent advances in digital health and artificial intelligence now offer promising tools to overcome these barriers.
Artificial intelligence can help identify high-risk patients by analysing large datasets from medical records and radiographs. Structured reports generated by AI algorithms can provide clinicians with patient-specific insights, supporting evidence-based recall planning and individualized risk communication.
In parallel, mobile applications are emerging as a new interface between clinicians and patients. Such apps can reinforce home-care instructions, track oral-hygiene behaviours, provide timely reminders, and motivate behaviour change—all tailored to the individual's needs.
The future of secondary prevention lies in combining professional expertise with technological innovation. By embracing AI-powered diagnostics and patient-centric digital tools, we can move towards truly personalized maintenance care that is not only more effective but also more engaging for the patient.
Biographies
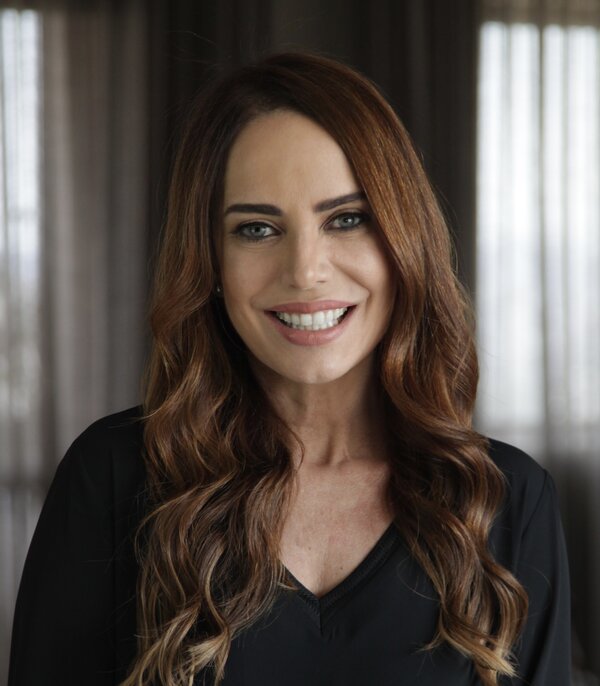
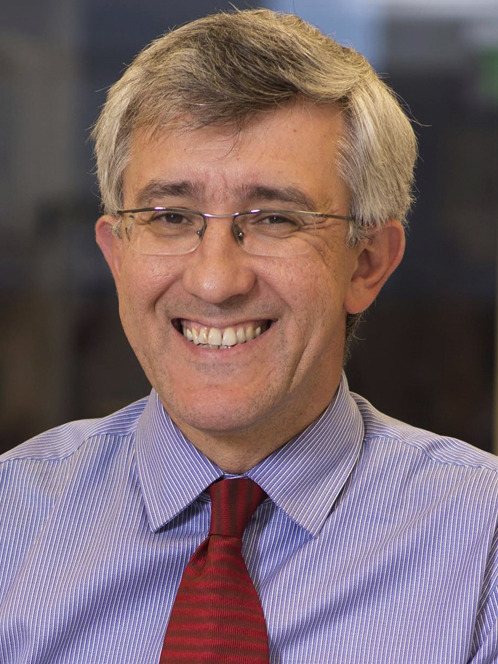
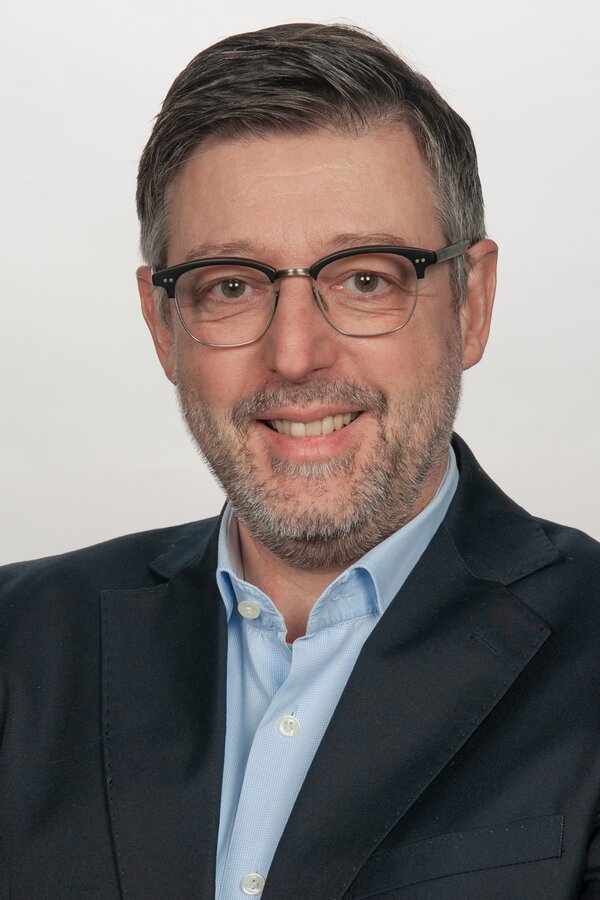
Magda Feres (DDS, MSc, DMSc) is the chair of the Department of Oral Medicine, Infection, and Immunity at Harvard School of Dental Medicine. With over 250 publications and 20,000 citations, her research—funded by FAPESP, NIH, and Harvard—has pioneered novel periodontitis treatments, investigated their effects on the oral microbiome, and advanced AI-driven personalized care. She has received prestigious awards, including the AAP Clinical Research Award, the IADR Women in Science Award, and the Harvard Medical School Dean’s Innovation Award for the Use of Artificial Intelligence.
Alpdogan Kantarci (DDS, PhD) is a Board-certified specialist of periodontology(C.A.G.S.) and a Diplomate of the American Board of Periodontology. He is the Erwin Schaeffer Endowed Chair, Professor with Tenure, and the Chair of the Department of Developmental and Surgical Sciences at the University of Minnesota School of Dentistry. He was previously a professor and senior member of the staff at the ADA Forsyth Institute, an associate professor at Harvard University School of Dental Medicine, and an associate professor at Boston University’s Henry Goldman School of Dental Medicine. He is the executve director of the International Academy of Periodontology and editor in chief of its scientific journal.
Christoph A. Ramseier (Prof. Dr. med. dent.) is a Swiss periodontist who received his education and training at the University of Bern. He earned his dental degree in 1995 and his doctorate in dental medicine in 2000. From 1999-2000, he underwent specialised training in periodontology and implantology at the University of Bern, where he became a board-certified specialist in periodontology by the Swiss Society of Periodontology (SSO/SSP) and the European Federation of Periodontology (EFP) in 2004. From 2004-2008, he was a research fellow at the University of Michigan, and in 2024 he became an associate professor in periodontology at the University of Bern, where his research focuses on periodontal risk management and supportive periodontal care.
EFP-IAP Joint Session: Personalized medicine – can we personalize periodontal treatment? EuroPerio11 | Vienna | Friday 16 May, 10-30-12.00
EuroPerio11 | Vienna | 14-17 May: Information and Registration