Therapy, Clinical & Translational Research, Article
Using AI to improve clinical decision-making in periodontology
11 April 2023
Research carried out at the EFP-accredited programme in periodontology at the UCL Eastman Dental Institute in London shows promising results on how artificial intelligence can be integrated into the clinical practice of periodontology to improve decision-making. Researcher Anastasiya Orishko explains the findings.
The 2017 World Workshop on the Classification of Periodontal and Peri-implant Diseases and Conditions brought significant changes for clinicians in everyday practice. This classification introduced multi-dimensional staging and grading and thus initiated a more complex decision-making process to reach a periodontal diagnosis.
The European Federation of Periodontology (EFP) developed teaching materials including videos and slide presentations, and published several decision-making algorithms and articles that aim to explain and clarify the new classification (Tonetti and Sanz, 2019). The federation has subsequently published clinical-practice guidelines on the treatment of periodontitis and, most recently, a series of infographics to help explain the step-by-step approach. Nevertheless — and perhaps surprisingly — clinicians still lack agreement in defining a “periodontitis” case (Marini et al., 2021).
In medicine, clinical decision-support systems (CDSS) have been widely implemented to aid the process of reaching a diagnosis. CDSS are tools in which patients’ characteristics are matched to a computerised clinical guideline to provide patient-specific recommendations or diagnoses (Sim et al., 2001). They have been promoted in healthcare for their potential to reduce medical error, increase efficiency, and promote evidence-based practice by adapting computerised clinical knowledge. There is evidence to suggest that 57% of studies on the implementation of CDSS had a significant impact on practitioner performance and 30% of them also on patient outcomes (Jaspers et al., 2011).
The aim of my MClinDent dissertation was to develop a CDSS to aid clinicians with periodontal diagnosis. The research project was led by Dr Federico Moreno and performed under the expert guidance of professors Francesco D’Aiuto, Ian Needleman, and Kenneth A. Eaton at UCL Eastman Dental Institute.
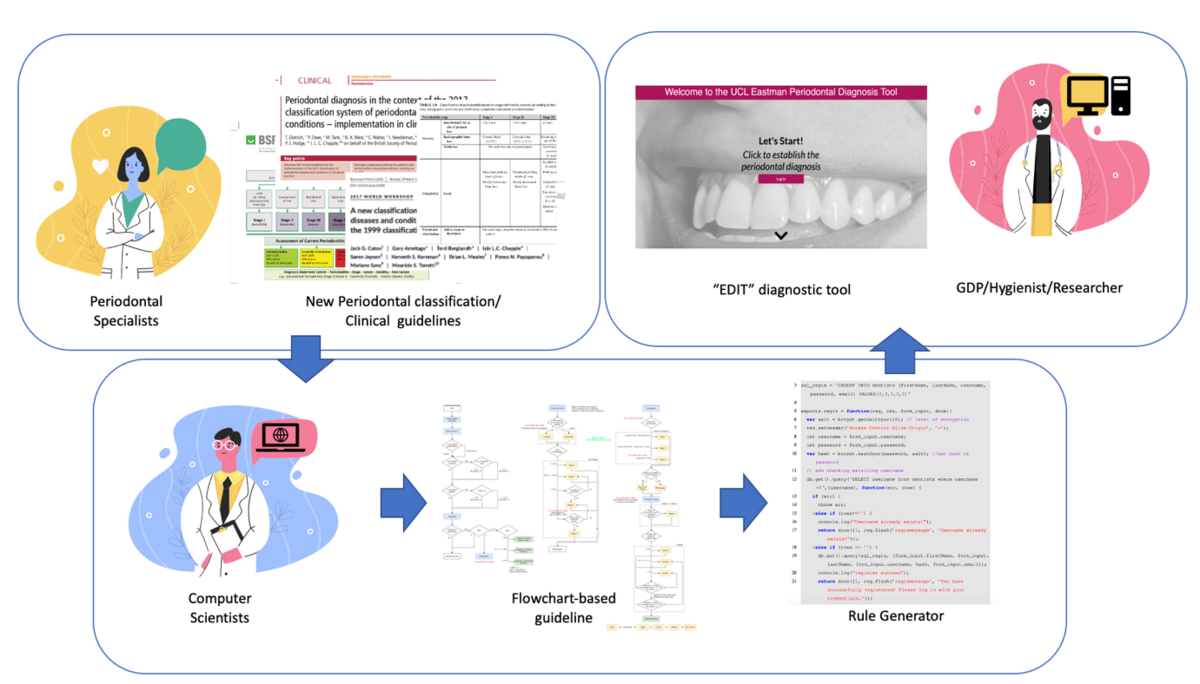
Our group envisioned a tool that would allow any clinician, regardless of their level of training and their location, to arrive at the same diagnosis for any given patient. The tool could be used not only for clinical purposes, but also for education, training, and calibration because it would ultimately aim to improve diagnostic precision and consistency among clinicians.
While planning the tool-development strategies, we realised that clinicians face an important challenge in terms of distinguishing between stage I and stage II periodontitis. The question arose: How reliably can one differentiate between bone loss of up to 15% of root length (Stage I) compared to bone loss extending from 15% to 33% of root length (Stage II)?
At present, the estimation of bone loss is done by visual assessment: the “eyeballing” of available radiographs. Inevitably, this can introduce a degree of subjective bias — especially in patients with an early stage of the disease — which may result in delayed treatment. Similarly, we can only diagnose rapid disease progression (Grade C) when signs of severe bone loss are clearly visible, as reflected in the bone loss/age ratio.
But what if you could measure the percentage of bone loss that has occurred within the past year or last six months in a matter of seconds? An additional aim of this project was to develop a built-in algorithm powered by artificial intelligence (AI) for the CDSS tool, which would automatically identify landmarks for bone detection and calculation on the periapical radiograph and then calculate the bone loss.
Methodology
This study was designed as an experimental development of the UCL Eastman Periodontal Diagnosis Tool (“EDIT”) with consequent validation. The project comprised two major components.
During the first part of the study, a close collaboration was established with the department of Computer Science at UCL. The periodontal diagnostic CDSS tool was developed based on the diagnostic logic produced by our research team using workflow-driven reasoning — a logic flowchart that directs the decision-making throughout the procedure — and rule-based reasoning (Figure 1).
Building the diagnostic logic was the most important and challenging part. For instance, the molar-incisor extent of periodontitis was not accounted for in the initial diagnosis flow chart. This prompted us to create a rule that was able to identify these specific cases. We therefore had to teach computer scientists and our CDSS system how to identify a case “in which at least two permanent teeth are affected, at least one of which is a first molar and no more than two teeth other than first molar and incisors” (Lang, 1999).
The initial iteration of the tool could not identify clinical periodontal health or periodontitis stability with a reduced periodontium. Therefore, one of the challenges we encountered was building an algorithmic logic behind the tool to be able to pick up probing pocket depths (PPD) and clinical attachment loss (CAL) values separately and generate a diagnostic output that accounted for both.
The identification of peri-implantitis cases also posed a challenge when building the application. The assessment of peri-implant health was the last functionality that we added to our tool and it demanded a completely separate logic that included all the details of the case definition described in the new classification by Berglundh et al., 2018.
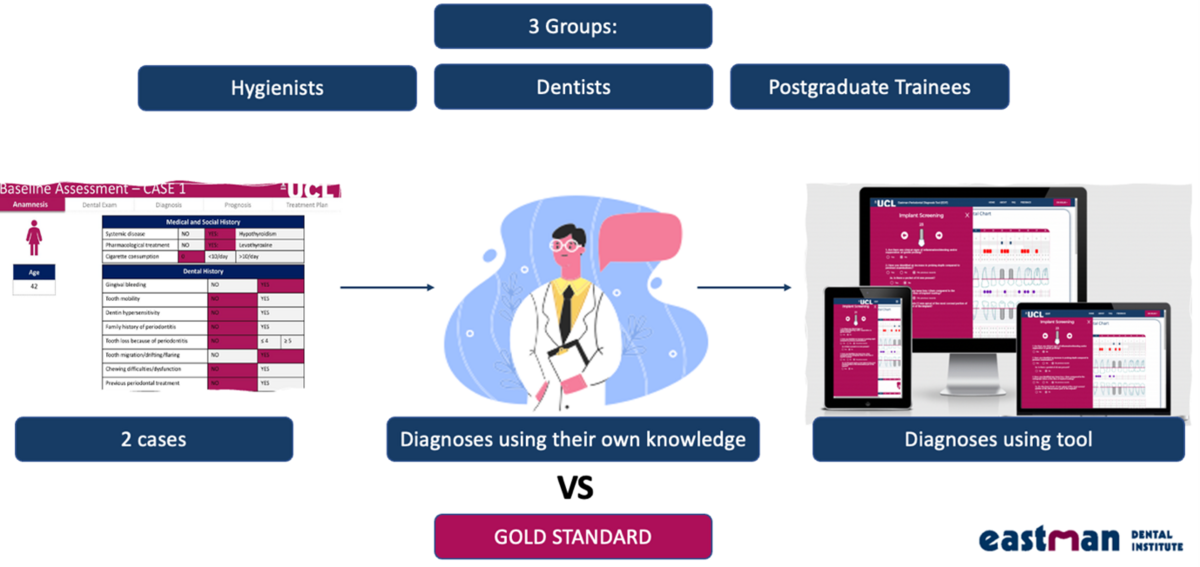
As the project progressed (along with several testing cycles), the structure of the diagnostic logic was continuously refined to ensure an accurate diagnosis using the tool.
The second part of the project was designed as a pre-implementation/validation study and consisted of the pilot testing of the tool by three focus groups (Figure 2). The aim was to compare the performance of the diagnostic tool, when used by clinicians, with the “gold standard”, defined as a reference diagnosis based on expert consensus.
The “gold standard” diagnosis for all 20 cases was reached and agreed on by the researchers (Anastasiya Orishko and Federico Moreno), according to the 2017 World Workshop definition (Tonetti et al., 2018) and the British Society of Periodontology’s implementation of the classification (Dietrich et al., 2018). Subsequently, advice was sought from the senior periodontal specialists who advised the team (professors Ian Needleman, Kenneth A. Eaton, and Francesco D'Aiuto). There were disagreements on the stage of six cases. These cases were discussed until consensus was reached. The resulting diagnoses were considered to represent the “gold standard”. This method to achieve a reference diagnosis has been shown to be feasible and acceptable in previous research (Handels et al., 2014) and has been applied in similar studies (Marini et al., 2020).
A database of 20 real-life cases with periodontitis with “gold standard” diagnoses was developed by the research team using anonymised data. The reliability of the results was then analysed using quadratic weighted Kappa statistics. Furthermore, we assessed the user acceptability and satisfaction with the tool. For this component, it was decided to use the validated system usability scale (SUS) (Brooke, 1996, Bangor et al., 2008).
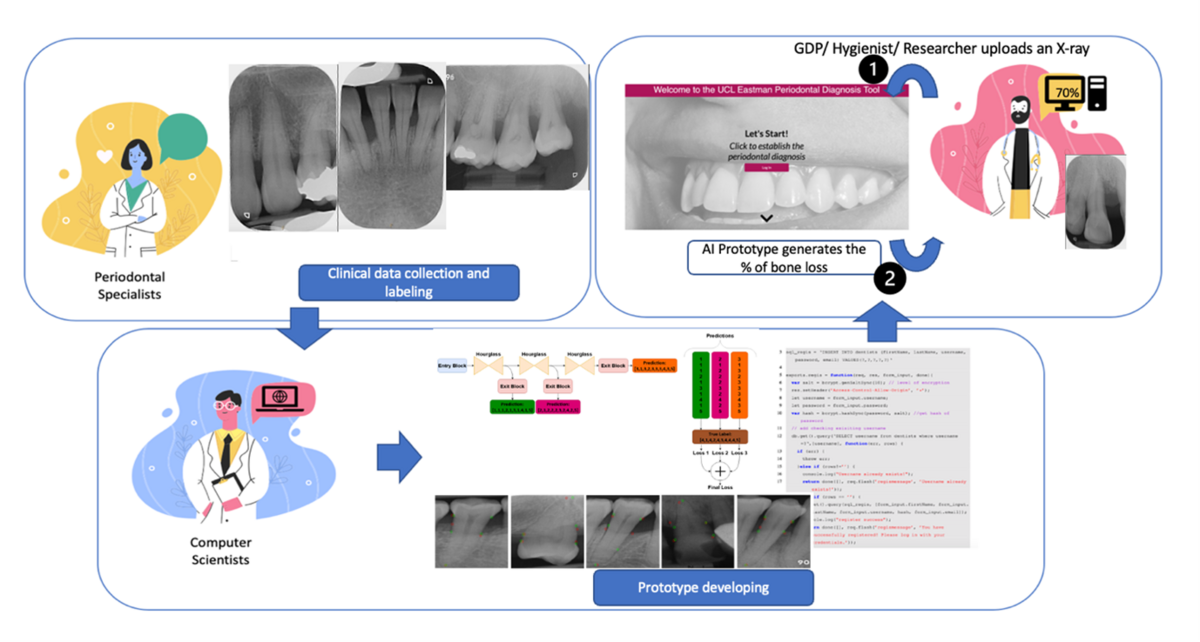
At the same time, a proof-of-concept study was undertaken in collaboration with the Welcome/EPSRC Centre for Interventional and Surgical Sciences (WEISS), at the Computer Science Department at UCL. This study resulted in the development of a novel model (neural network regression) using 340 annotated X-rays of teeth (Figure 3). Various landmarks for calculating bone loss were marked on the X-rays by a panel of clinical experts: a) cementoenamel junction (CEJ), b) bone levels, and c) the apex for each root, also outlining each tooth and bone region. Next, an AI neural network was adapted (based on Tiulpin et al. 2019) and trained to recognise these landmarks and estimate bone loss accordingly (Figure 4).
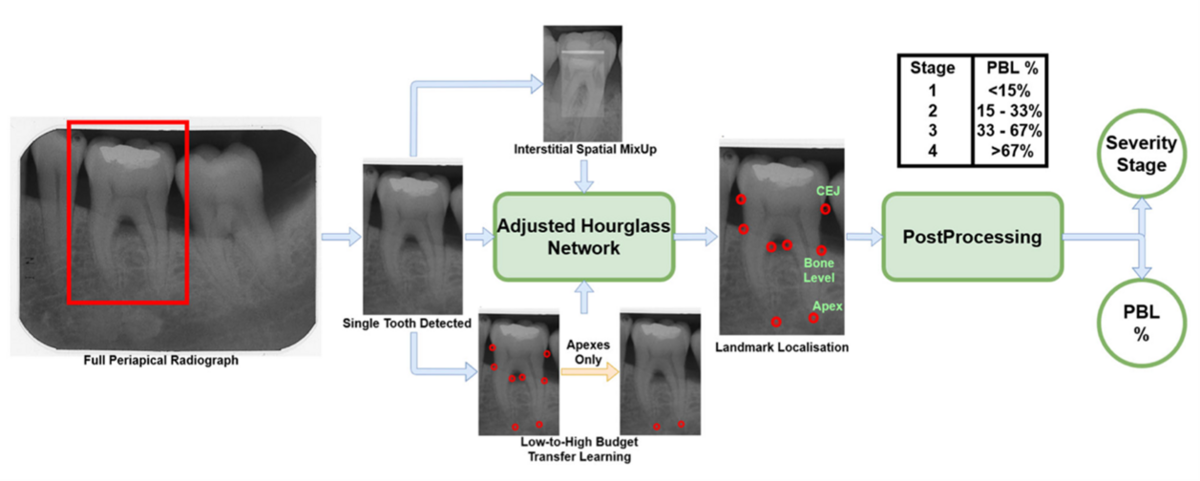
Results
The tool-validation pilot study demonstrated statistically significant agreement between tool-derived diagnosis and the gold standard. Agreement between the three focus groups and the gold standard varied from moderate to substantial when participants relied on their own knowledge. When study participants were asked to use the tool to reach a diagnosis, the agreement increased to substantial and almost perfect levels (statistically significant). This was further accompanied by an excellent usability score (overall SUS = 82).
It is interesting to note that when the tool was used to inform clinicians on the extent of periodontitis, it always demonstrated the highest agreement, but when we focused on staging and grading there was a much greater variability. It can be speculated that these observations result from the objective assessment of disease extent using a formula integrated into the tool’s algorithm and from the fact that the staging and grading of periodontitis depend on the calculated percentage of bone loss indicated by the clinician and modelled against the correct age of the patient.
These results might suggest that perception and estimation of the percentage of bone loss at the worst-affected site in a case of periodontitis will vary between clinicians. Similarly, during the establishment of the gold-standard diagnoses, disagreement arose within the expert group only when staging was completed — indeed, this topic needed to be resolved by discussion.
It might be hypothesised therefore that subjective perception of bone loss at the worst-affected sites, when measured by visual examination of radiographs, does introduce bias into the diagnostic process. This is an important finding, because the assessment of the radiographs of patients with periodontitis is critical for adequate diagnosis and current methods of interpretation might lack sensitivity (Tonetti et al., 2018).
The results of our proof-of-concept study were peer-reviewed and published, underlying the potential of using AI for objective bone-loss measurements (Danks et al., 2021). The performance of landmark detection for single-rooted teeth showed encouraging results (>80% accuracy), which prompted us to progress to a larger dataset collection and the further development of the proposed algorithm.
Small data sets (fewer than 1,000 units per group) have been yielding an accuracy of less than 90%, with 4,095 samples per group needed to reach the “desired” accuracy of 99.5% (Cho et al., 2015, Hwang et al., 2019). This helped us shape the next stage of validation of this tool including three different groups of teeth: single-, double-, and triple-rooted teeth.
To achieve sufficient diagnostic sensitivity, a sample size of at least 3,000 periapical radiographs would be required. Therefore, we are now working on further tests of the tool’s effectiveness in a larger validation study, after addressing the required improvements that were identified in the pilot study. Our aim is to partner with industry for the AI model to be linked with the CDSS tool as part of a clinical-management software suite.
This project could represent the way forward to personalised periodontology and would allow the tailoring of prevention, treatment, and supportive care based on each patient’s needs. Automation of periodontal bone-loss assessment and calculation using an AI-based tool can be considered a paradigm shift towards computer-assisted healthcare.
Federico Moreno adds: “We have been working on this project for some time and Anastasiya played an integral part. Machines are able to make objective measurements on images more accurately than the human eye and are also able to process large amounts of complex data much more efficiently than humans. Our project provides a perfect example of how we will be able to incorporate AI and computer decision-support systems into our clinical practice in the future.”
Select bibliography
Bangor A, Kortum PT, Miller JT. 2008. An Empirical Evaluation of the System Usability Scale. International Journal of Human–Computer Interaction, 24, 574-594.
Brooke J. 1995. SUS: A “quick and dirty” usability scale. Imprint CRC Press.
Danks RP, Bano S, Orishko A, Tan HJ, Moreno Sancho F, D’Aiuto F, Stoyanov D. 2021. Automating periodontal bone loss measurement via dental landmark localisation. Int J Comput Assist Radiol Surg.
Jaspers MW, Smeulers M, Vermuelen H, Peute LW. Effects of clinical decision-support systems on practitioner performance and patient outcomes: a synthesis of high-quality systematic review findings. J Am Med Inform Assoc, 18, 327-34.
Marini L, Tonetti MS, Nibali L, Rojas MA, Aimetti M, Cairo F, Cavalcanti R, Ferrarotti D, Graziani F, Landi L, Sforza NM, Tomasi C, Pilloni A. 2021. The staging and grading system in defining periodontitis cases: consistency and accuracy amongst periodontal experts, general dentists and undergraduate students. J Clin Periodontol, 48, 205-215.
Sim I, Gorman P, Greenes RA, Haynes RB, Kaplan B, Lehmann H, Tang PC. 2001. Clinical decision support systems for the practice of evidence-based medicine. J Am Med Inform Assoc, 8, 527-34.
Tonetti MS, Sanz M. 2019. Implementation of the new classification of periodontal diseases: Decision-making algorithms for clinical practice and education. J Clin Periodontol, 46, 398-405.
Tiulpin A, Melekhov I, Saarakkala S. 2019. KNEEL: knee anatomical landmark localization using hourglass networks. CoRR abs/1907.12237.
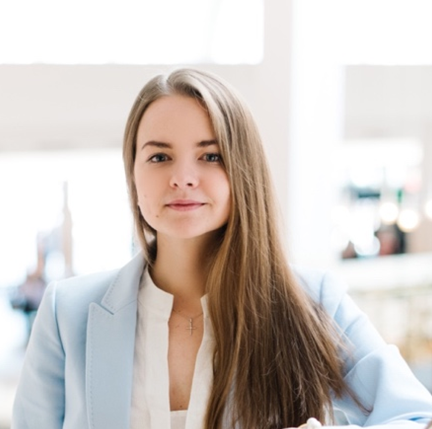
Anastasiya Orishko obtained her dental degree with honours in 2013 in Ukraine. Inspired by Professor Niklaus Lang’s lectures when she was a dental student, she developed a passion for periodontology and decided to move to London to pursue her career further. She has dedicated herself to a full-time three-year specialist training in periodontology at the UCL Eastman Dental Institute in London and graduated in 2021. Anastasiya currently combines clinical activity in private practice with teaching at UCL Eastman.